Building Artificial Intelligence on Health Data
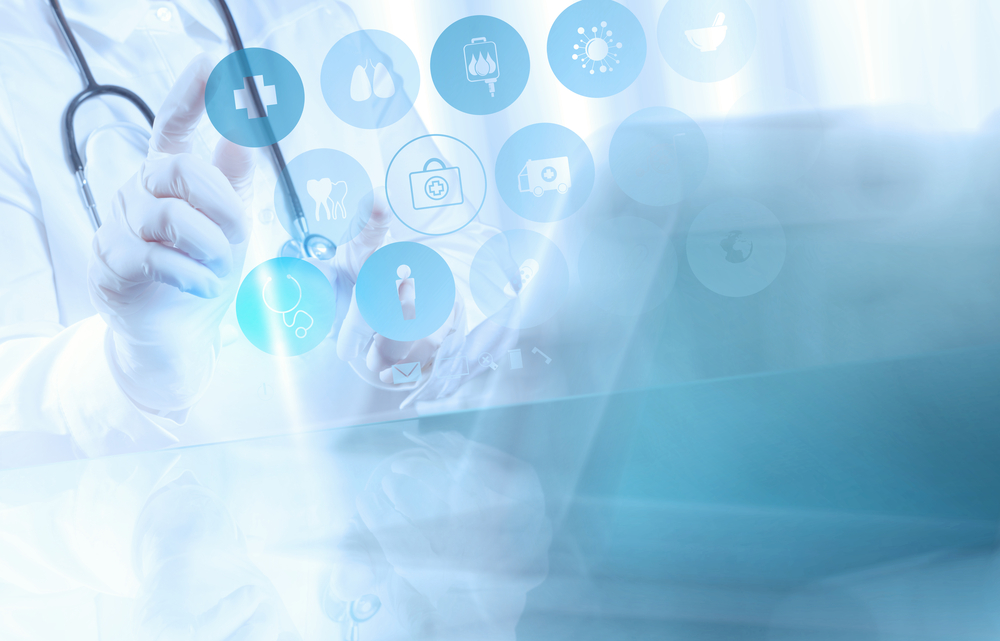
- Artificial intelligence is built on strong data strategies, but exchanging protected health information through an EHR system is a challenge.
- Electronic health records are restricted by privacy compliance and low device interoperability.
- Technology companies are developing ways to collect and apply data throughout clinical workflows.
Health technology can provide efficiency solutions for orthopedics, to help meet the growing demand
Treatment for musculoskeletal injuries and deformities often includes orthopedic surgery, and the demand is growing rapidly. The total number of orthopedic procedures performed annually – including repairs, corrections, and joint replacements – is expected to double over the next two decades.
Surgeons will burnout from increased working hours, hospitals will struggle as operational inefficiencies scale, and device manufacturers will exhaust profits while scrambling to manage inventory. Patients will face waitlists spanning a year for elective procedures – unless they are wealthy, of course. For populations like the middle class, the middle-aged and elderly who are suffering from osteoarthritis will go untreated. The current system will collapse when burdened with twice the patient volume, unless the industry prepares by building technology to allow for more patients to be treated using less time, money, and effort. However, digital and accessible clinical notes, clinical decision support, and predictive models can be utilized with AI to help alleviate this problem.
Advanced technology like artificial intelligence will transform orthopedic care
Medical device research has been moving away from basic implants, instruments, devices, and moving towards advanced computers, programs, and software. This new wave of health technology focuses on more powerful systems that will increase operational, financial, and clinical efficiency. This is why device manufacturers are collaborating with technology companies to innovate using emerging technologies like artificial intelligence. Orthopedics is already a $50 billion global industry – a good reason for technology companies to make investments in this specialty.
Enhatch has a vision for orthopedic care and health technology called Intelligent Surgery™: the use of artificial intelligence to enhance implant design, expedite surgical technique, optimize inventory logistics, and simplify clinical workflows by connecting and learning from data during each step of the surgical care continuum.
Artificial intelligence is built on health data, but access to health data is limited.
Artificial intelligence is not pixie dust to sprinkle onto current systems and magically fix them. Trends like artificial intelligence and machine learning have not taken quickly to health markets because they require a solid data strategy first.
A data strategy is the framework for collecting data and using data. With the emergence of data science, the goal is cross-modality analytics – that is, collecting health data from various sources and normalizing the data into quantified, usable formats. This includes turning large volumes of patient records into searchable databases and auditing billing codes for missed reimbursements.
Although healthcare produces enormous volumes of variable information at a high velocity – the three V’s of big data that artificial intelligence thrives on – the information is not freely exchanged. Health data is very difficult to access, due to high privacy concerns and low interoperability.
Privacy concerns dictate how patient data is stored and used.
An individual patient generates hundreds of thousands of data points, if not millions in their impact on the greater system. Most of this information is stored in Electronic Health Records (EHRs), which were mandated by the government as part of the Health Insurance Portability and Accountability Act (HIPAA). HIPAA also set requirements for healthcare professionals to have policies that protect patient information. Protected Health Information (PHI) includes medical history, visit records, health status, insurance claims, forms of payment, and billing information – all that could be linked to the individual.
One part of HIPAA regulations is storing health data in physically secure locations. This means only authorized health professionals can access hardware that contains records – like computers and other devices. This ensures that the data is accurate, only to be viewed and changed by authorized personnel. Part of data storage protection involves backing up the EHR system in case of loss or theft.
Another part of HIPAA compliance is minimum sharing of health data in secured methods. This means only parties with permission should be able to access networks that contain records – like software programs and online portals. This requires specialized Information Technology (IT) departments to setup safeguards like firewalls to prevent breaches. An important part of data sharing protection involves encryption, which put information into an uncodable form when sending, so that third parties do not maintain private health data.
De-identified health data is one approach to building artificial intelligence.
To design an artificial intelligence layer for health data strategies, health data can be de-identified. This means that certain metadata fields of files are cleared of anything that can be linked back to the individual. This happens before the files are sent out through the firewalls of the hospital’s system.
These anonymized data sets are sent to technology companies who are developing the software. They are used for training purposes, to build and validate artificial intelligence systems, but actual patient health information is used one the system is adopted. Using machine learning, the quality improves over time as decisions and outcomes are tracked.
Some health professionals believe that the physician can decide appropriate sharing, others believe that the patient must consent to contribute their information to research. The opt-in paradigm ensures that individuals controls their information and maintain ownership of their data, rather than the data aggregator.
A lot of health data is trapped, unretrievable even with permission.
While HIPAA compliance limited permission to store and use health data, the protections lead to low technology communication and integration, trapping health information.
Older EHR systems weren’t designed to share information because the exchange wasn’t important or allowed. This is why we see medical equipment full of legacy systems – outdated devices that do not have compatible file formats or the means to send files. viewers, adapters, and converters are still needed for communication between systems.
The idea of plug-and-play interoperability, medical devices that can be physically linked together to exchange information with each other, is only possible for an average of three devices in a room. Exchanging health information still largely depends on human effort to manually coordinate and transfer data.
Standards like Health Level Seven (HL7) and Digital Imaging Communications in Medicine (DICOM) were created to increase file compatibility, and Application Program Interfaces (APIs) are used to integrate services. Still, there are many challenges to collecting health data. Some technology companies are developing new ways to bridge legacy systems to cloud-based platforms, though there has been much pullback on that appro.
The best way to collect and apply health data has yet to be discovered.
In an ideal world, information would flow seamlessly and invisibly throughout the healthcare ecosystem. One authority system would orchestrate information exchanges and monitor authorizations.
However, all of the computer systems can not be redesigned or replaced. Health technology will remain heavily fragmented for some time, while technology companies solve challenges to EHR integration and PHI security.
At Enhatch, we are developing new ways to collect and apply health data from end-to-end across the surgical care continuum for orthopedics.
Our cohesive Intelligent Surgery™ Platform offers a planning application for surgeons that connects with an inventory management system for device manufacturers.
As soon as a surgery is scheduled, the system retrieves the patient’s images. The algorithm automatically generates a patient specific 3D model, and uses the bone predictive models to size and position the implants. The surgeon reviews the surgical plan and approves of the order. The inventory management system receives the request and the device manufacturer fulfills the order. This clinical workflow is simplified with a continuous and seamless flow of information.