Intelligent Spine Surgery: Improving Accuracy through Predictive Analytics
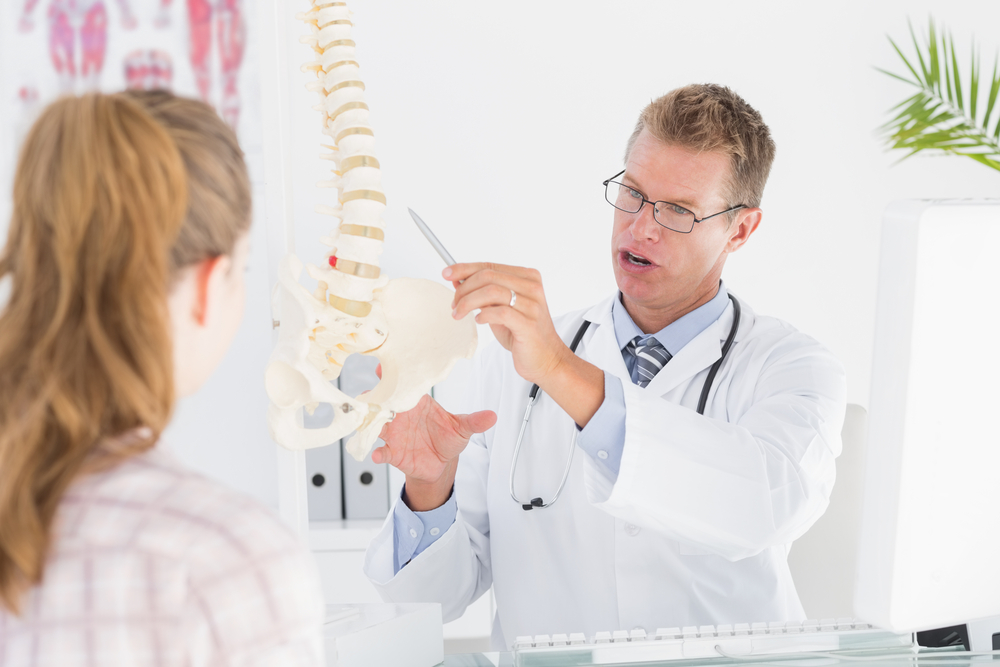
Artificial Intelligence (AI) is expected to save US Healthcare $150 billion annually by 2026. Connected technologies, preliminary diagnosis tools, and intelligent assistants are among the top ten drivers. For surgeons, AI-powered predictive analytics can help understand preoperative risk, increase efficiency, and improve patient outcomes.
The world of spine surgery is no different. Spine surgery is complex. Surgeons need to make several key decisions right from the start. These considerations include:
- Can the patient be treated without surgery or is surgical intervention required?
- Is the patient a candidate for Minimally Invasive Surgery (MIS) or do they need traditional open spine surgery?
- Are there any high-risk factors that may negatively impact patient outcomes or result in a readmission?
- How is the bone condition? Can I use a traditional Off-the-shelf (OTS) implant or a patient specific implant (PSI)?
Additionally, the entire healthcare industry is gearing towards achieving greater efficiencies without compromising on accuracy. Surgical caseloads are on the rise due to an aging population but pricing continues to drop. There is also higher demand from payers and patients for more procedures in an outpatient setting, where applicable. This trend is already gathering steam for hip and knee arthroplasties.
Predictive Analytics in preoperative planning can help surgeons:
- make decisions faster,
- understand risk, and
- improve patient satisfaction to meet the needs of the changing surgical landscape.
Understanding Preoperative Risk to Optimize Postoperative Outcomes
Spine surgeries are complex procedures. Preoperative conditions and each patient’s health history greatly impact postoperative outcomes. For elderly patients, understanding risk at a preoperative stage becomes even more critical. This is because they are more likely to have one or more comorbidities.
This is where AI and Machine learning can help. Predictive analytics can help us analyze vast amounts of historical patient data to accurately predict patient risk and outcomes based on preoperative conditions.
Using AI, surgeons can get a risk-reward profile of each patient before surgery. This insight can be used to educate patients on the lifestyle or health habit changes required that can help their recovery. For example, imagine a heavy smoker about to undergo surgery, or patients with chronic comorbidities like diabetes and hypertension. Factoring this information into analytical models can help guide medication protocols and help counsel patients accordingly.
Another instance where AI can be of help is to help refine pain management for lower back surgery. Non-invasive, non-drug options are often the first step in management of lower back-pain. But prescription opioids are also used as a last resort for patients suffering from prolonged lower back pain. One challenge when using opioids is a risk of certain patients developing a longer-term dependence on opioids. Predictive analytics can help healthcare professionals identify patients at a higher risk of continued long-term opioid dependence. Surgical planning and medication protocols can be adjusted accordingly.
AI can also help reduce avoidable readmissions to hospitals within 30 days of surgery - a key healthcare quality metric. Avoidable readmissions impact patient satisfaction, outcomes, and healthcare costs. Avoidable readmissions across healthcare procedures are estimated to cost the US healthcare system a staggering $17 billion annually. In the world of Spine surgery, infections and refractory pain are some common reasons for unplanned readmissions. Better post-discharge care is a key step that can be used to reduce some of these unplanned readmissions.
By taking into account various pre-discharge variables, historical readmission data, pre-existing conditions, and postoperative data, AI can help surgeons predict the risk of readmissions for their patients.
Let us look at Adult Spinal Deformity Surgery (ASDS). This is an example of a surgery with higher complication rates. Studies show that ASDS has an estimated 30-day readmission rate of 7.5%. It is often difficult for surgeons to predict post-operative outcomes in such surgeries. But by using AI-based predictive analytics, surgeons can get unique insights into potential patient outcomes and help reduce the risk of readmission.
Increased Accuracy Through Better Preoperative Planning
AI can help surgeons better understand each patient’s diseased anatomy, and reduce inefficiencies in the surgical preop planning process.
After analyzing hundreds of thousands of patient medical scans, AI can generate accurate, personalized 3D anatomic models for each patient. This can help surgeons prepare for surgery, educate patients and caregivers on what to expect during surgery, and potentially improve surgical accuracy.
The emergence of 3D printing as a technology to generate patient-specific implants from AI-based models is also a game changer.
Patient-specific implants (PSI) offer the potential for better clinical outcomes in unusual and complex cases compared to traditional off-the-shelf (OTS) implants. In such cases, there could be an implant-bone shape mismatch with traditional OTS implants. This mismatch would need to be compensated through more remodeling of the bone. This could increase surgical time and weaken the bone structure. In such cases, PSI may be a better solution since they are customized to the patient’s particular joint anatomy.